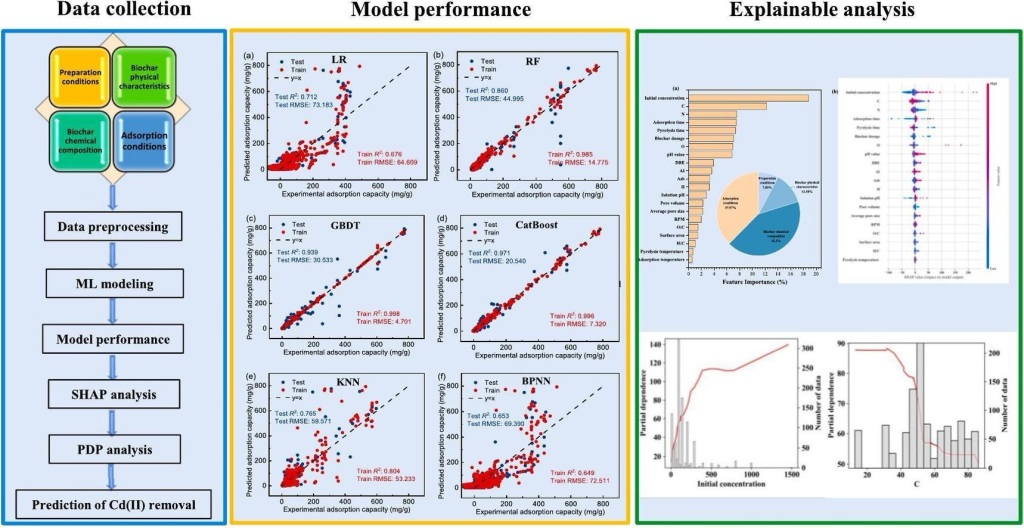
Recent research in Science of The Total Environment explores the use of machine learning to enhance the efficiency of biochar materials in removing cadmium (Cd(II)) from wastewater. The study compared six machine learning models: Linear Regression, Random Forest, Gradient Boosting Decision Tree, CatBoost, K-Nearest Neighbors, and Backpropagation Neural Network. Among these, the CatBoost model demonstrated the highest predictive performance for Cd(II) adsorption capacity.
The research utilized a comprehensive dataset comprising 1051 data points, factoring in variables like biochar preparation conditions, physical and chemical properties, and adsorption experiment settings. The CatBoost model outperformed others with a test R² value of 0.971 and a low root mean square error of 20.54 mg/g.
Feature importance analysis using Shapley Additive Explanations (SHAP) highlighted that the chemical composition of biochar was the most significant factor influencing adsorption capacity, accounting for 42.2% of the impact. Adsorption conditions followed closely at 37.57%, with physical characteristics and preparation conditions contributing less significantly.
Optimized conditions for maximum Cd(II) adsorption were identified through partial dependence plots. These conditions include a high initial Cd(II) concentration, specific carbon and nitrogen percentages in biochar, a prolonged adsorption time, and precise biochar dosages and pH levels.
This study offers new insights into biochar’s role in Cd(II) adsorption and provides a robust framework for designing biochar materials for effective wastewater treatment, leveraging advanced machine learning techniques to optimize environmental remediation efforts.
Leave a comment